What Is The Role Of Dataset In Machine Learning?
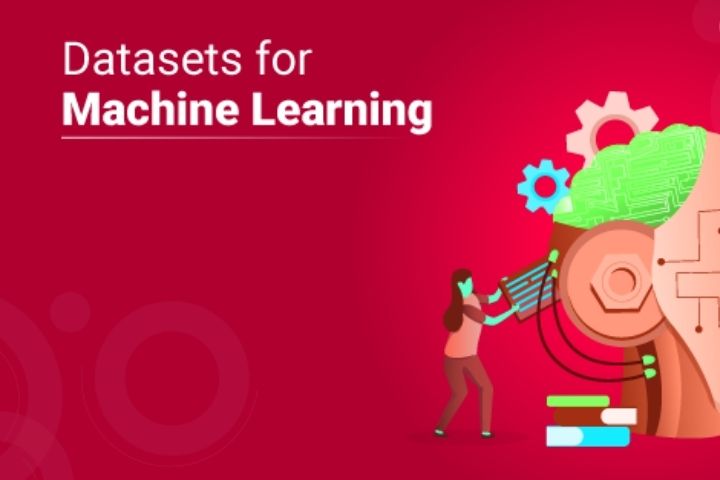
Are you aware of the technicalities involved in making Machine Learning models holistic, intuitive, and impactful? If not, you first need to understand how each process is broadly segregated into three phases, i.e., Fun, Functionality, and Finesse. While the ‘Finesse’ concerns training ML algorithms to perfection by first developing complex programs using relevant programming languages, the ‘Fun’ part is all about making the customers happy by offering them the perceptive and intelligent fun product.
However, nobody talks at length about the ‘Functionality’ bit of the process, which mostly involves data preprocessing techniques and basics of data collection, data annotation, and more. And intertwined with these methods and techniques is something that data and ML experts tag as Datasets.
In the subsequent sections, we shall touch upon every aspect of a Machine Learning Dataset by first understanding the basics and advanced concepts and dependencies relevant to the same and second, delving deep into the benefits and examples for a more accommodative stance towards the subject.
Table of Contents
What Is A Dataset In Machine Learning- Everything That Matters?
Let’s go by the book first. An ML dataset is perceived as a single entity by the algorithms despite housing disparate chunks of data. And each dataset is fed into the system to train the algorithm into finding the predictable patterns housed within, as the dataset in principle is more of a collection, comprising separate chunks of usable data.
And data is arguably the most essential component of any AI or ML model as every business needs to keep historical customer behavior in mind and train their models accordingly. This approach helps them build a product that is proactive and highly analytical. Also, customer behavior is highly erratic, and therefore, truckloads of data and corresponding datasets need to be fed for the models to become more comprehensive and holistic over time.
Importance Of Data In Machine Learning
So datasets and the corresponding data chunks are meant for training, right! Well, not exactly, as data in Machine Learning serves multiple purposes. While training ML algorithms is the key element, lending ‘Finesse’ to the models by validating the training set and even testing the prepared model are also made possible with relevant data.
Therefore, the next time you plan on connecting with an experienced data collection and annotation service provider, be sure of the fact that they procure datasets for a wide range of tasks and even split the same to suit model requirements.
How To Prepare A Dataset?
Now that you have established the premise relevant to datasets, it is important to know more about preparing them for perfection. And even though, as a business, you might never need to get behind the preparatory logistics, it is better to keep up with the process.
Experienced service providers follow a set format to prepare relevant datasets, which include:
- Data Collection– Via web scraping, open-source access, public AI repositories, and other relevant avenues
- Preprocessing– Reappropriating the collection data by cleaning the same and making it model-specific
- Annotating– Data within a dataset needs to be labeled for the machine to understand it better, and this is what annotation is all about
How Is The Quality Of A Dataset Determined?
If you are concerned about the quality of data fed into the system, make sure it adheres to the following pointers:
- Relevance
- Coverage
- Validity
- Completeness
- Accessible
- Quality-specific requirements
- Quantity-specific needs
- Analyzed or not
- Connected or not
And unless the datasets adhere to these prerequisites, they cannot be termed as high-quality training datasets. Also, even if the collection of data is on point, inexperienced dataset creators often end up goofing up preprocessing and annotation, which eventually impacts the quality of the AI model.
Example Of Dataset In Machine Learning
Unsure as to which data chunks qualify as datasets? For starters, anything that is being researched, collected, preprocessed, and annotated by an experienced AI and ML service provider as per your model-specific requirements qualifies as a dataset.
It can either be relevant audio files to train NLP models, dictation notes and verbatim text files for healthcare offerings, written and spoken notes in different languages to prepare conversational AI models, and more.
However, if you want to find your own datasets, the Google dataset repository comes with several reliable public datasets, including the ones from Kaggle, VisualData, CMU Libraries, and more. And if you want to get a better understanding of the type of datasets, there are geographic datasets, housing datasets, computer vision datasets, NLP datasets, and more.
Wrap-Up
If you plan on building an efficient Machine Learning model in the future, it is important to get the hang of datasets in play. Even though you might still need a credible AI-specific firm to get hold of those algorithmic-relevant datasets, it is better to get a clear understanding of how the entire process works. And, most importantly, even though there are several public datasets available, it is important to ensure that they adhere to the quantity and quality standards before they can even be used to train ML models to perfection.